1. Introduction
In this study, we propose two novel CPI prediction models SgCPI and SgCPI-KD for unseen proteins: 1) SgCPI for the inductive settings, semi-inductive settings and transductive setting, and 2) SgCPI-KD for more accurate CPI prediction in the inductive setting. SgCPI integrates the inter-interaction network and the intra-physicochemical features of query proteins and compounds with a sampled subgraph-based heterogeneous graph neural network (HGNN). To further enhance the prediction performance of discovering novel compounds for novel proteins, SgCPI-KD designs a knowledge distillation strategy to transfer the knowledge of known CPIs to novel molecules. SgCPI-KD designs a MLP student model to estimate potential neighbors of query molecules from the molecular representations generated by the HGNN teacher model SgCPI, then SgCPI-KD combines the message of potential neighbors and the physicochemical features of query molecules to enhance the CPI prediction between novel proteins and novel compounds. The framework is shown in Figure 1.
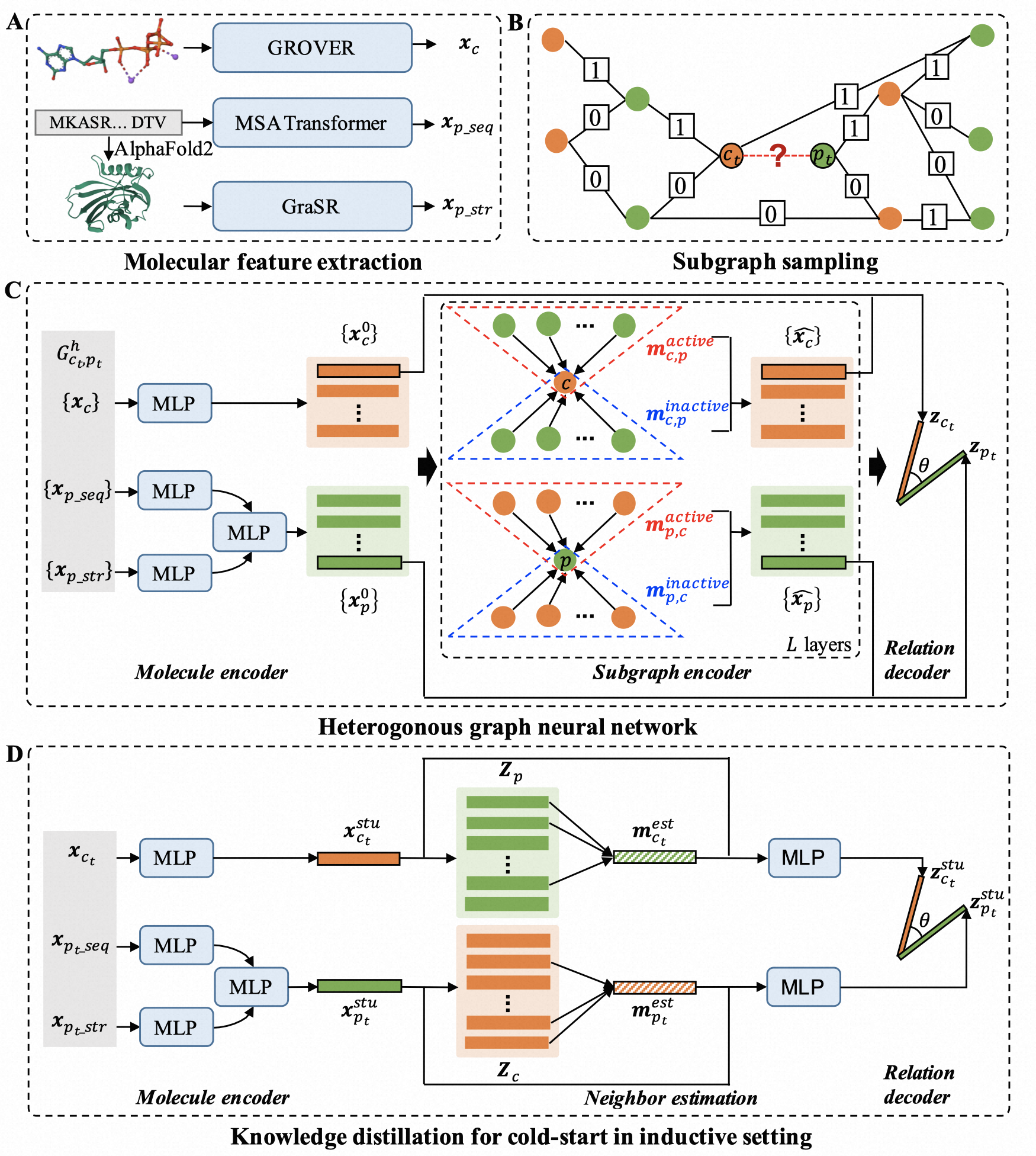
2. Input
First, for predicting ligand-binding residues, please input the protein chain structure (in PDB format)
and 1-character chain ID (chain ID is case sensitive. If your query chain doesn't contain chain ID, just leave the chain ID box blank).
Then, please input the compound SMILES.
For a submitted job, if there's only one query compound, it takes about five minutes for prediction, and each additional query compound increases the running time by 30 seconds. We will send the results to your email when the job is finished.
3. Output
We will send the results to your email when the job is finished.
Results will be shown in the result page (example) when the job is finished. In addition, results can be downloaded by clicking "Download results".