iLocator: An Image-based Multi-label Human Protein Subcellular Localization Predictor
Introduction
Human cell is organized into compartments of different biochemical cellular processes. Being at the right place at the right time of proteins is critical for normal cell functions. Protein mislocalization is one of the typical features of cancer biomarkers. To reveal these cancer-related mislocalizations, we have developed an image-based multi-label subcellular location predictor called iLocator, which covers 7 cellular localizations, i.e. cytoplasm, endoplasmic reticulum, Golgi apparatus, lysosome, mitochondria, nucleus, and vesicles. The iLocator incorporates both global and local image descriptors, and uses an ensemble multi-label classifier to generate accurate predictions. It is featured by the capability of dealing with both single and multiple location sites proteins. Total 3240 normal human tissue images with known subcellular locations from the Human Protein Atlas (HPA) were used to train and test iLocator. And then by using constructed iLocator, we performed predictions for 3696 protein images from 7 cancer tissues, which have no location annotations in HPA. By comparing the outputs of normal and cancer tissues, we detected 8 potential cancer biomarker proteins having significant localization differences with p-values less than 0.01.
The flow chart of the whole experience is shown in Figure 1.
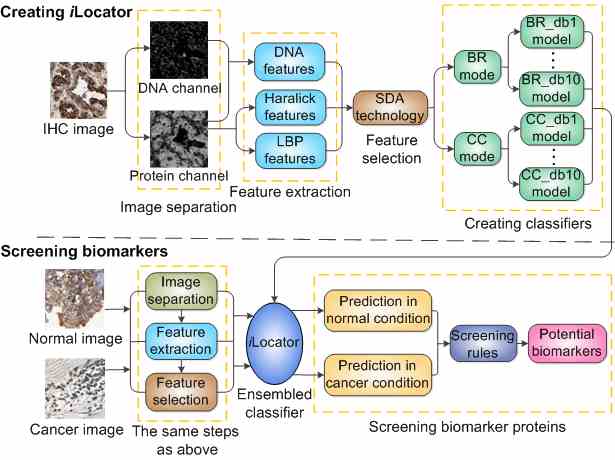
Fig. 1. The flow chart of creating iLocator and screening biomarkers.
Software
The interface of the iLocator software is shown in Figure 2. The software can work at two modes. Mode1 can output the prediction of images in the given path, and Mode2 can output the predictions of images in normal and cancer paths and compare these two conditions.
If you have installed Matlab 2011a under Windows operating system, please click 32bit or 64bit to download the software.
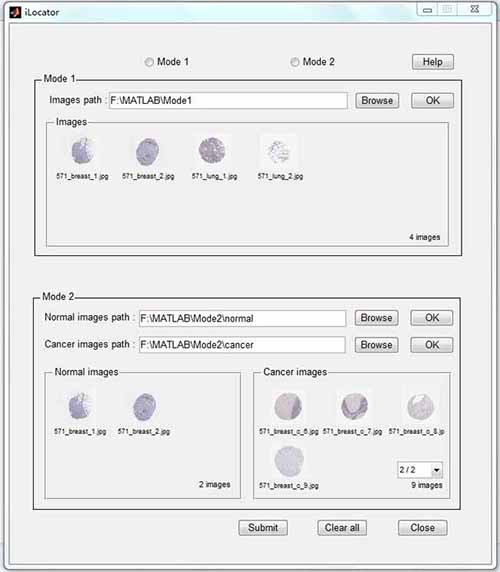
Fig. 2. The interface of iLocator.
Code and dataset
The data and code are contained in the following compressed files:
Click here to download the image dataset (5.21Gb), and click here to download the source code for academic use only (313Kb). The code package has been tested using Matlab 2011a under Windows 7 in a 64bit architecture.
High-resolution figures in the paper
Fig 1. The flowchart of the experiment
Fig 2. The results of determining the threshold T
Fig 3. The results when adding LBP into feature space
Fig 4. The comparison of results in the single-label and multi-label testing dataset
Fig 5. The protein mislocalizations detected by the iLocator
Link
Hum-mPLoc: An online amino acid sequence-based human protein subcellular location predictor.
Reference
Ying-Ying Xu, Fan Yang, Yang Zhang, Hong-Bin Shen, An image-based multi-label human protein subcellular localization predictor (iLocator) reveals protein mislocalizations in cancer tissues, Bioinformatics, 2013, 29: 2032-2040. [download the Supplementary data]
|
|